English headline: Can Machine Learning Improve Predictions Effectively?
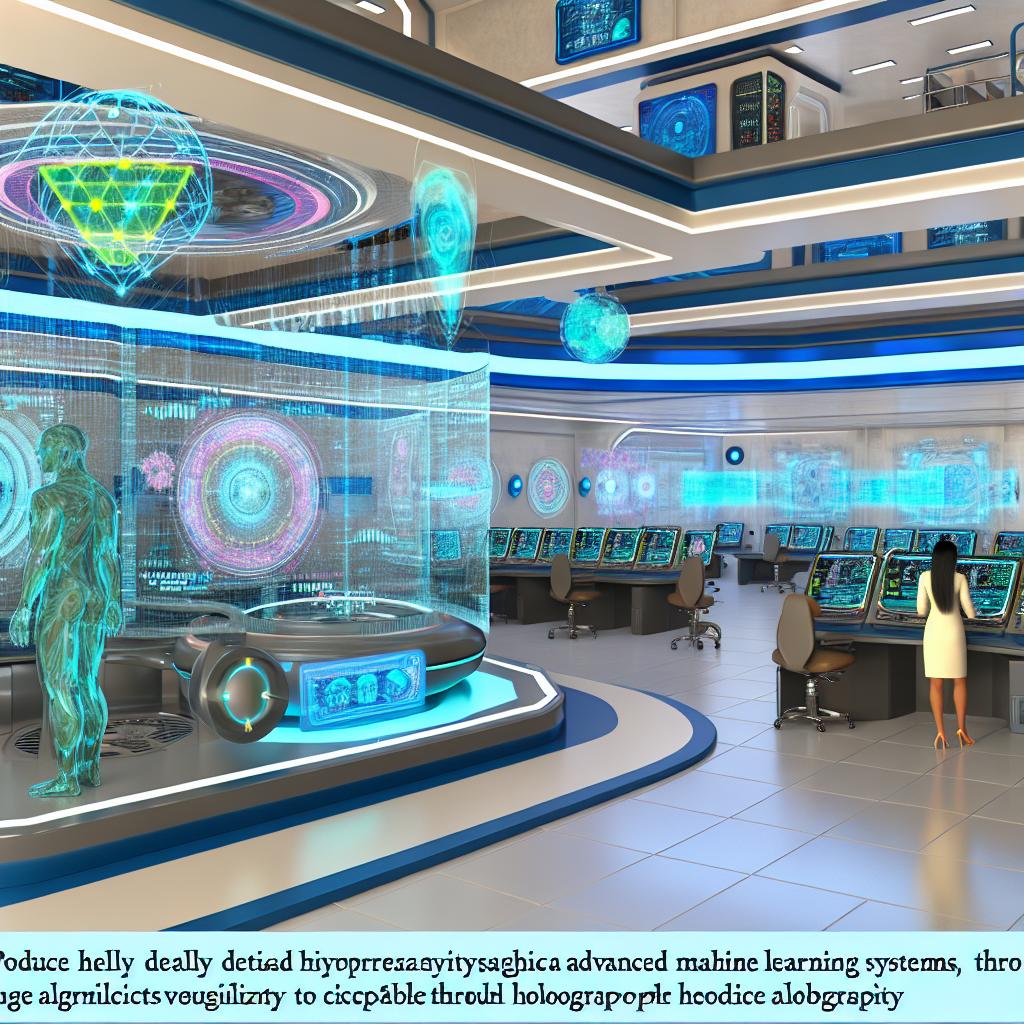
- Machine learning improves prediction accuracy by finding patterns in large data sets.
- Key fields include finance, healthcare, and sports for faster, confident decision-making.
- Algorithms like decision trees, neural networks, and support vector machines enhance accuracy.
- Real-time data processing is crucial for timely predictions.
- Supervised and unsupervised learning improve predictions via different methodologies.
- Reinforcement learning allows systems to learn through rewards/penalties.
- Predictive analytics tools like RapidMiner, SAS, and IBM SPSS process big data for insights.
- Challenges include data quality, model complexity, and ethical issues such as bias and privacy.
- Emerging trends involve self-supervised learning, responsible AI, and quantum computing.
- AI enhances decision-making in healthcare and education; future forecasting technologies guide industries.
Can machine learning transform the way we make predictions? Let's dive into this groundbreaking technology that might redefine predictive accuracy. As an avid tech fan, I unravel how algorithms and real-time data drive precision. With a focus on technological trends and the venture capital markets, this exploration will open new doors for those eager to learn and invest in forward-thinking tech companies. Curious yet? Let’s explore!
How Does Machine Learning Improve Prediction Accuracy?
Machine learning can seem complex, yet it plays a big role in our daily lives. It helps enhance prediction accuracy across various fields, making forecasts more reliable. This section will explore how machine learning achieves this and the real-world impact of its growing influence.
What is Predictive Machine Learning?
Predictive machine learning is about using computers to predict outcomes. It examines patterns in huge amounts of data, finding trends and relationships. This technology helps businesses make decisions faster and with more confidence. It finds use in finance, healthcare, and even sports. For example, in healthcare, it can predict patient outcomes based on historical data. In finance, it can forecast stock trends or credit risks. By learning from past data, machine learning provides insights that are hard to detect manually.
How Do Algorithms Enhance Prediction Accuracy?
Algorithms serve as the backbone of predictive accuracy. They provide rules for machines to analyze data and improve predictions. Some key algorithms include decision trees, which resemble a flowchart to decide predictions. Another is a neural network, which mimics human brain processes. It is great for complex tasks like image recognition. Support vector machines can classify data points into different categories. Each algorithm suits different types of data and prediction needs. By using the right algorithm, machine learning systems improve their accuracy significantly.
Why is Real-Time Data Processing Critical?
In today's world, data changes rapidly. To keep predictions accurate, processing data in real-time is crucial. Let's say you're using a navigation app. It needs to adjust your route when traffic changes. This happens thanks to real-time data processing. Technologies like stream processing and distributed computing enable this swift data handling. With them, systems crunch data as it's created, ensuring the latest information guides your predictions. This leads to timely and relevant outcomes, especially vital in fast-paced sectors.
Predictive machine learning doesn't stop at better accuracy. It's transforming how industries operate by making informed decisions easier. Thanks to machine learning, we can tackle challenges once thought too complex for traditional methods.
How Are Machine Learning Algorithms Demystified?
Machine learning, with its vast applications, fascinates me profoundly. These computer systems can learn from data and make accurate decisions. Let me guide you through how these smart algorithms work in predictions.
What is the Role of Algorithms in Prediction?
Algorithms act as the brains of machine learning. They find patterns and use them to make AI-driven forecasts. There are many algorithm types, each with its own strengths. Let me focus on a few that often shape AI forecasts.
One type is decision trees. A decision tree splits data into groups. It asks a series of questions to reach a decision. For example, the weather forecast often uses decision trees to predict rain or sunshine.
Another is neural networks. These mimic the human brain. They consist of 'neurons' that pass data through layers to learn complex patterns. Neural networks are used in voice assistants, like telling a flashlight from a camera flash.
Support vector machines are crucial, too. They draw lines that separate data into classes. This method helps distinguish between different patterns. For instance, sorting spam emails from inbox ones relies on support vector machines.
Each of these key algorithms contributes to impressive AI outcomes and forecasts. By combining their strengths, AI models can solve many problems.
How Do Supervised and Unsupervised Learning Differ?
Supervised and unsupervised learning are two main models in machine learning. Both help in predictive modeling, but they do so differently.
In supervised learning, the algorithm is like a student with a teacher. The algorithm learns from labeled data. For example, it knows photos are of cats if labeled as cats. Supervised learning can predict new, unlabeled data, like identifying new cat photos.
Unsupervised learning has no labels to guide it. Picture an explorer finding patterns in unknown lands. Here, the algorithm discovers hidden structures in data. For instance, it might group similar customer behaviors without being prompted.
Both methods improve predictions, but in unique ways. Supervised learning builds from known patterns, while unsupervised discovers new ones.
Can You Explain Reinforcement Learning Strategies for Predictions?
Reinforcement learning is like training a puppy with treats. It's a strategy where systems learn by getting rewards or penalties.
In industry, I see reinforcement learning in action, especially in game AI. When AI plays a game like chess, it learns with each move. If it wins, it gets a ‘reward,’ boosting its method.
Another example is in autonomous vehicles. Here, the car learns how to navigate roads. If it avoids obstacles successfully, it gets positive feedback. Each journey enhances its driving skill.
Reinforcement learning strategies are changing how we innovate. They empower AI to learn like living beings, leading to smarter systems that improve over time.
Machine learning continues to impress with its capability to predict and adapt. Different algorithms and strategies unravel new potentials and precision in forecasts.
What are Predictive Analytics Tools and Their Applications?
Predictive analytics tools are like crystal balls for businesses. They help us forecast the future by finding patterns in data. When combined with machine learning, they become smart tools that give even better predictions. Let me walk you through some of the most effective tools, the role of big data, and how this works across different industries.
Which Are the Most Effective Predictive Analytics Tools?
There are many tools that help with predictive analytics. Some of the most popular ones are RapidMiner, SAS, and IBM SPSS. Each tool offers unique features that cater to various business needs.
RapidMiner is known for its user-friendly design. People love it for its deep learning and real-time data processing abilities. It also allows us to build models without coding – perfect for those who do not write code.
SAS is another powerful tool. It has been in the predictive analytics world for a long time and offers many features like advanced graphics and detailed statistics. It is popular in medicine for patient data analysis.
IBM SPSS helps us with text analysis and hypothesis testing. It's perfect for survey data and market research.
These tools use advanced techniques to process and analyze huge amounts of data. They turn raw data into valuable insights. This means businesses can use these insights to make smarter decisions. Choosing the right tool depends on what a company needs, such as cost, ease of use, or special features.
How is Big Data Integrated into Predictive Models?
Big data plays a huge role in predictive analytics. It gives us a lot of information, sometimes too much to handle. But with proper methods, big data helps us make better predictions. It gives a full picture and lets us see trends and patterns.
Handling big data can be hard. It needs special techniques and tools to break it down into useful parts. We must first clean the data, removing errors or irrelevant parts. Then we organize it so algorithms can work their magic.
One challenge is storing big data. It requires many resources and can be costly. However, cloud computing helps by providing space and power to process large data sets. Another challenge involves privacy. With more data, the risk of exposing sensitive information grows.
Despite these hurdles, the power of big data in enhancing predictive models is strong. Businesses use it not just to predict but also improve their products based on foresight. If one can manage its integration well, big data becomes a game changer in predictive analytics.
How Do Predictive Modeling Techniques Vary Across Industries?
Different industries use predictive modeling in unique ways. Each industry tailors its techniques based on specific needs and data types. Here's how various sectors harness this power.
In Healthcare, predictive modeling helps improve patient care. It predicts health trends, helps in developing new medicines, and can even foresee outbreaks. Techniques used involve analyzing patient histories and genetic information to offer personalized treatments.
Retail heavily relies on predictive analytics to forecast demand and manage inventory. Predictive models help businesses understand customer behavior. For example, online stores use it to recommend products based on past purchases.
Finance uses these models to assess risks and prevent fraud. Predictive models predict stock trends, assess loan applications, and monitor market changes. Such models analyze past transactions to ensure stability.
Manufacturing industries use predictive maintenance models. These models predict machine failures before they happen. This minimizes downtime and reduces costs.
By customizing the techniques per industry, predictive modeling offers specialized insights, ensuring businesses stay one step ahead. It allows for precision in planning and provides valuable foresight, essential in today's fast-changing world. Each sector refines their approach to cater to their unique data challenges and opportunities.
Using predictive analytics and machine learning, we give businesses the tools to see tomorrow's opportunities and challenges. These insights can turn potential threats into new avenues for growth.
How Does Data Influence Predictive Learning?
Data is the core of effective predictive learning. It shapes and guides machine learning models toward accurate predictions. Let's explore how data selection, refinement, and mining affect predictive learning.
How Crucial is Selecting Appropriate Data Sets?
Choosing the right data set is very important in predictive learning. A good data set ensures high accuracy and model reliability. If you pick the wrong data, your predictions might fail. In machine learning, data informs the model's learning process. For instance, if a model predicts weather, using data from a hot desert won't work to predict a rainy jungle climate. The Stanford Question Answering Dataset (SQuAD) method helps us evaluate how well our model understands and predicts real-world situations. This method relies on precision, ensuring predictions use the most accurate and complete data. Good data must always be recent, related, and real.
What Are Techniques for Refining Predictive Accuracy?
Once the data set is chosen, refining this data becomes essential. One way to sharpen accuracy is feature engineering. This involves creating new features or modifying existing ones to enhance the learning experience of a model. For example, if predicting a student's grades, adding features such as study hours, past scores, and class attendance might improve accuracy. Another method is data refining techniques. This involves cleaning the data, removing errors, and ensuring quality. Practice involves removing duplicate information, fixing missing values, and normalizing the data. By cleansing the data, we improve its purity, and models then make better predictions.
How is Data Mining Linked to Predictive Analytics?
Data mining is a process to identify valuable patterns in large data sets. It’s a crucial part of predictive learning. Data mining involves exploring huge data repositories and spotting trends, which help in making informed predictions. It supports the creation of data-driven predictions by uncovering hidden insights.
A key benefit of data mining is its ability to manage big data integration. With data mining, predictive models identify and use only the most relevant information. This allows the model to function efficiently without being bogged down by irrelevant data. However, challenges exist in mining data. The task of sifting through large data sets can be taxing and requires proficient tools and skills. Yet, when done correctly, data mining improves predictive model accuracy and reliability.
Understanding data's role in predictive learning is vital to harness its full potential. By carefully selecting data sets, refining techniques, and aptly utilizing data mining, we develop models that predict precisely and powerfully. Data drives these models, making predictions more reliable and applicable in real-world scenarios.
What Challenges and Ethical Considerations Exist in Predictive Machine Learning?
Predictive machine learning promises to transform the way we see the future. But, it is not without its problems. The goal is to make accurate predictions. Yet, implementing these models comes up against several challenges. This section looks at these hurdles, the ethical issues we must address, and how real-world data plays a role in predictive analytics.
What Are Common Challenges in Implementing Predictive Models?
Building predictive models is not easy. They come with a mix of technical and operational hurdles. One key challenge is data quality. Without good, clean data, predictions can be way off. Models need data that is accurate, up-to-date, and complete. Gathering and securing this data can be hard. Also, bad data can lead to wrong conclusions.
Another issue is model complexity. Predictive models often use math and computing power that can be hard to understand. When things get too complex, errors become more likely. This can also make it hard for people to trust the model's output.
To beat these challenges, teams must focus on building strong processes. First, they must make sure data is cleaned and verified. Training the model on various data can also help it adjust and get better. Simple models are sometimes as good as, or better than, very complex ones. Testing models in different settings can show their strengths and weaknesses and help improve them.
What Ethical Considerations Should Be Addressed?
Ethical considerations in AI predictions are crucial. Machines can make choices based on patterns they see, but do these choices hurt people? AI sometimes carries our biases. If we use past data that reflects bias, models may repeat these unfair patterns.
Let's also think about privacy. Machines need lots of data, some of it personal. Protecting people's privacy while using this data is vital. There must be clear rules about what data can be used and how.
Another ethical issue is transparency. People should know how AI reaches its decisions. If a model can explain its choices, it's easier to trust the results. This is why having a clear ethical framework is important. Guidelines assist in making sure AI's predictions are fair.
How is Real-World Data Utilized in Predictive Analytics?
Using real-world data sounds simple, but it brings its own set of challenges. Real-world data is messy, full of gaps, and often not standard. It needs lots of work to clean and organize it so that models can use it well. This involves several processes like data cleaning, normalization, and integration.
Yet, the richness of real-world data can make predictions strong. When models consider data from various areas, like health, markets, or weather, predictions get better. For example, Google Maps uses real-world data to help users navigate cities more easily and safely. They use AI to provide info about traffic, road conditions, and even the best lane to be in.
The key is in how you handle and use this data. By learning from the ways companies successfully use data, others can follow suit and also succeed. Models that accept wide-ranging data inputs are set up for innovation.
In conclusion, predictive machine learning is a shining field full of chances. It also has its set of obstacles and ethical questions. Solving these will lead to better, more accurate models that can help everyone. Our job is to face these challenges with clear plans and an ethical approach that puts people first.
What Are Future Trends in Predictive Machine Learning?
What Are Emerging Trends in Machine Learning?
Machine learning (ML) never stops evolving. Right now, new trends are changing what it can do. One big trend is making ML smarter. This means creating models that learn on their own without much help. Some call this self-supervised learning. It saves time and effort because we don’t need to label tons of data by hand.
Another trend is responsible AI. Here, ML models explain their choices. They show us why they make certain predictions. This trust feature helps people understand and accept AI decisions better.
Quantum computing is another game-changer. It's still in the early days, but it could make ML super fast. Imagine crunching massive amounts of data in a blink. This could make predictions quicker and more accurate.
Let’s not forget about AI fairness. Researchers work hard to remove bias from ML systems. They aim to make AI fair for everyone, no matter their background. Companies invest in this to ensure their models treat everyone equally.
These trends are shaping the future of ML. They're not just ideas. They're steps toward smarter, more inclusive machine learning.
How Will AI-Powered Decision Making Evolve?
We see machines making choices all around us. They help pick your shopping list or suggest movies. But AI-powered decision-making is set to change even more.
In the future, expect AI to make quick decisions in complex jobs. Think of doctors diagnosing illnesses faster with AI help. Or imagine teachers getting AI tips to improve how they teach each student.
AI will also get better at understanding how people feel. It will listen to our voices and read our faces. This human-like understanding means machines can guess when we’re happy, sad, or angry and help us better.
Moreover, businesses will lean on AI for decisions more. They’ll rely on AI to find trends and predict market changes. This means staying ahead and meeting customer needs before they arise.
All this shows that AI won't ever replace people but will assist them. It promises smarter decisions and improved support in every area of life.
What Is the Impact of Future Forecasting Technologies?
Technology for predicting the future is growing. These technologies help us see what might happen tomorrow. Google Maps' updates give us a peek into this future. For instance, it uses AI to suggest places to visit. It predicts what users may want to know about locations before they ask.
Another tech, augmented reality (AR), brings virtual images into the real world. Apps like Google Maps use it to give directions. This method is called Immersive View. It helps people find landmarks and avoid hazards better.
Voice recognition tools are improving too. They're like having a chat with your phone. On-the-go users can report traffic issues with a quick word. This speeds up how fast information gets shared and acted on.
Future forecasting will shape industries far and wide. Healthcare, transport, and entertainment all stand to benefit. As these forecasting tools grow, they'll help us make more informed, efficient decisions.
Predictive machine learning stands on the brink of transformation. Trends today promise to refine and broaden the scope of everyday tools. The innovations also highlight our constant journey toward smarter, fairer, and more insightful machines.
Conclusion
Machine learning is transforming how we make predictions. We've explored predictive machine learning, the role of algorithms, and the magic of real-time data processing. Predictive learning is powerful, especially when using the right tools and data. Yet, challenges and ethical concerns demand attention. As we look to the future, emerging trends promise even smarter predictions. With the right mix of tools and ethical practices, machine learning will reshape industries and open new opportunities. Let's embrace this journey and continue exploring the ever-evolving world of AI and technology trends.